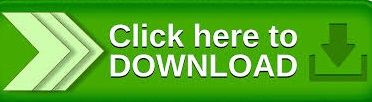
- #CHIANG ELEMENTS OF DYNAMIC OPTIMIZATION PDF FILES FULL#
- #CHIANG ELEMENTS OF DYNAMIC OPTIMIZATION PDF FILES CODE#
Our results suggest that on average the overhead of profile collection can be reduced by half (SPEC95) to almost two-thirds (SPEC2000) relative to the Ball-Larus algorithm with minimal impact on the information collected. Its increased efficiency comes from two sources: (i) reducing the number of potential paths by not enumerating paths with cold edges, allowing array accesses to be substituted for more expensive hash table lookups, and (ii) not instrumenting regions where paths can be unambiguously derived from an edge profile. is an extension to the Ball-Lams efficient path profiling algorithm.
#CHIANG ELEMENTS OF DYNAMIC OPTIMIZATION PDF FILES CODE#
This notion of profile-guided profiling is a natural fit for dynamic optimizers, which typically optimize the code in a series of stages. The key idea to our approach, called targeted path profiling (TPP), is to use an edge profile to simplify the collection of a path profile. However, maximizing profits in a highly competitive cloud market.

For cloud providers, the typical goal is to maximize their profits. We present a technique for reducing the overhead of collecting path profiles in the context of a dynamic optimizer. As the demand for computing resources grows, cloud computing becomes more and more popular as a pay-as-you-go model, in which the computing resources and services are provided to cloud users efficiently. The framework proved to be a valuable tool for students to easily obtain guidelines for both the model aircraft design and control to maximize the competition score.

#CHIANG ELEMENTS OF DYNAMIC OPTIMIZATION PDF FILES FULL#
The wing area should be increased within allowable limits to maximize payload capacity, climb to maximum target height should be the focus of the first 60 s of flight and full throttle should be avoided in cruise to reduce losses and extend flight distance. The results revealed the need to consider the multi-objective total score to account for the different score components and highlighted the importance of the payload level and chosen trajectory. The optimization problem totaled 206 variables and 283 constraints and was solved in less than 7 h on a standard computer with 12% reduction when using b-splines for trajectory control variables.
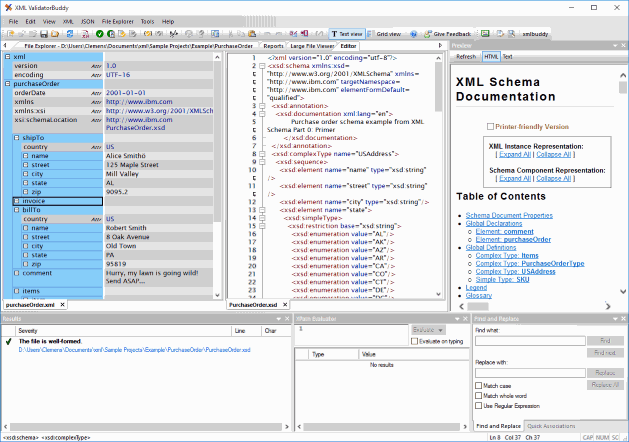
A trajectory optimization module using a collocation method is coupled with the option of using b-splines to increase computational efficiency together with an experimentally-based power decay model that accurately determines the aircraft propulsive response to control input depending on the battery discharge level. It is based on OpenAeroStruct, an aerostructural solver that uses analytic derivatives for efficient gradient-based optimization. Its modular architecture makes it easily adaptable to any problem where the performance depends not only on the design of the aircraft but also on its flight trajectory. A coupled aerostructural aircraft design and trajectory optimization framework is developed for the Air Cargo Challenge competition to maximize the expected score based on cargo carried, altitude achieved and distance traveled.
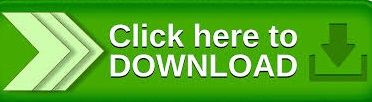